Beyond the hype: Is a 10x leap in efficiency possible with AIOps in IT observability?
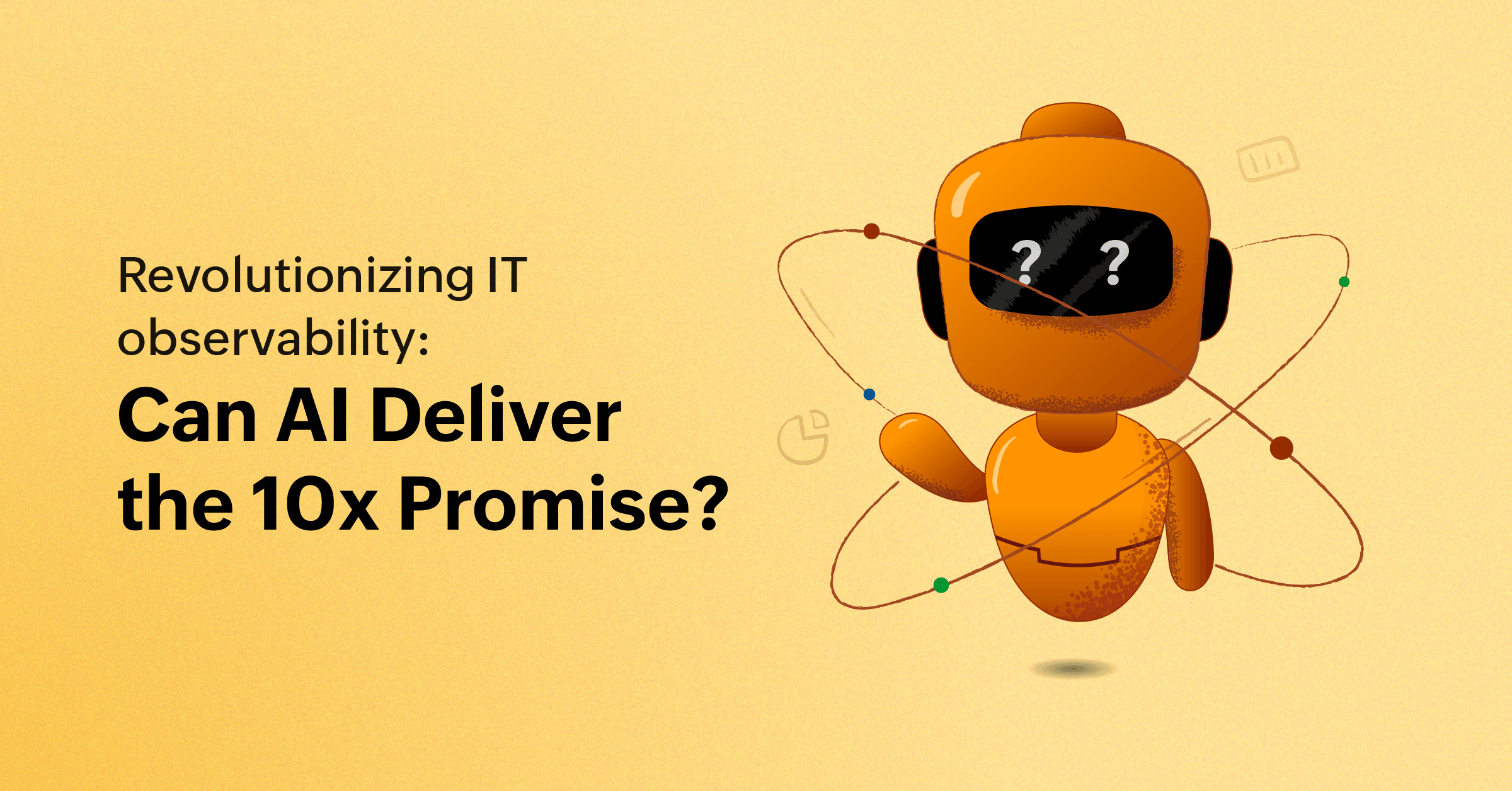
Now that AI has revolutionized IT forever, what are its implication on IT observability? Typically, IT operations, SREs, and DevOps professionals use IT observability to gain a holistic view of their IT infrastructure. In that pursuit, they used AIOps in several ways. Now, AI has helped IT observability with better anomaly detection, faster root cause analysis, and proactively identifying opportunities to dynamically scale IT to ensure uptime, performance, and security.
Six ways AIOps now helps in IT observability
Automation: AI in IT observability can instantly automate mundane, repetitive tasks such as restarting servers, clearing caches, trigger certain workflows, or even proactively expand capacity to match oncoming traffic. This also helps ensure compliance and security, as AI can enforce best practices in a larger, more dynamic IT landscape compared to what humans can achieve.
Anomaly detection: AI sees with more than two eyes (metaphorically speaking) and surpasses human inadequacies, blind spots, lapses, or shortsightedness to look into multiple sources of data to unearth anomalies better. By engaging ML algorithms, AI can sift through logs, metrics, and traces to unearth patterns or deviations and predict issues like an impending massive failure much ahead and clearer than traditional monitoring systems where intricate signals could sometimes go unnoticed.
Resilience through faster resolution: With AI, root cause identification gets more rapid and sharper, and so does the mean-time-to-resolution (MTTR). AI can correlate incidents across systems and suggest possible solutions based on historical data—by matching it with past incidents to perform course correction. All these benefits of AI help a company establish high standards in product quality that will be heard and appreciated by the customers.
No false alerts: AI excels in its ability to discern false positives in monitoring signals and cuts such noise by counter-checking other factors across the system before flagging true anomalies. This ability starts with a minimum data set and gets better with time as AI learns the subtle art of discerning genuine threats while ignoring benign events—like a temporary and allowable spike in CPU usage, etc. This frees up time for IT teams to focus on systemic improvements.
Adaptive, not alarmist: With AI, nothing is written in stone. It adapts to emerging changes and dynamically adjusts the thresholds for alerts based on a variety of factors, including operational context, seasonal trends, or specific load-based demands, to ensure that monitoring alerts are relevant and yet not alarmist. The adaptive ability of AIOps in IT observability is crucial for IT teams to maintain performance without constant reactionary or anxiety-ridden tweaks.
Proactive infrastructure management: Across your IT infrastructure, AIOps take a proactive approach by constantly predicting demand and identifying inefficiencies to suggest changes or even effect automatic adjustments. AI scales your IT dynamically, optimizes network resources to handle changing traffic, or even reroutes data flow to make your IT remarkably resilient.
Quantifying the breakthroughs made possible by AIOps in IT observability
Scalability in data: AI can crunch a greater volume of datasets with high variations in its 3Vs—volume, velocity, and variety—to offer unprecedented, instant insights into what is beyond a human's reach. In doing so, AI has significantly accelerated the responsiveness of modern IT operations.
Boost in productivity: AIOps-led productivity boost can increase a team’s productivity by helping eliminate incidents through automation and better alerts. Let us assume that in a mid-sized IT organization, an incident takes two hours to resolve, and an average of 100 events happen per month, that totals to 2,400 incidents per year. This time saving is more than the 2,080 hours of hours per employee per year, in a 40-hour work-week format.
Improvement in service quality: IBM found that AIOps-led observability can help reduce 50% of incidents, 80% time spent wasted in addressing false positives, and reduce time to fix issues by an impressive 75%.
Ability to save costs: AIOps, by eliminating manual intervention at many levels, boosts productivity for all team members. AIOps can give you substantial cost savings, along with the implied benefits of using your existing workforce more effectively, where it matters.
Great strides, indeed, but it is also important to weigh these five challenges to factor in the hype about AI in IT observability.
Complexity: IT environments continue to be complex, and AI integrating seamlessly across diverse systems is tough to handle and requires skilled personnel. Data quality issues and integration hiccups are on the way. As cloud-native technology evolves, AI-led solutions should evolve too and ensure there are no drops in the quality of actionable insights and automation potential derived from it.
Comprehensibility: Considering the foundational work needed in data cleanup, modern legacy rationalization, and robust training for all staff, AIOps in IT observability can yield exponential benefits only when its role is comprehensively integrated across the organization.
Credibility: AI could inherit biases in training data, leading to skewed or unfair outcomes. In observability, where decisions are based on instant insights, any misreading could affect system performance. Teams need to find ways to ensure algorithmic fairness. Also, some AI decisions could be in the dark owing to their "black box" nature, and systems that could lead to explainable choices are needed.
Culture: The transition to AI-driven systems demands cultural and operational changes within organizations, which can only be achieved with rigorous training and reimagining of workflows to set realistic expectations and establish checks and balances.
Continuity: The AI model must be continuously trained to scale the benefits exponentially, and that requires constant involvement from a skilled team.
Cost: AI models demand high costs, too, as they run on energy-intensive infrastructure and are enabled by large organizations driving key changes. This calls for leadership that can effectively transition their IT operations to derive the full benefits of AI by designing the objectives and workflows.
The way forward
AI in IT observability, particularly with platforms like ManageEngine Site24x7, holds profound promise for enhancing efficiency. However, while the goal of a 10x productivity increase captivates the imagination, the current state of technology and organizational readiness suggests a more measured expectation.
The journey towards such a goal involves not just technological advancements but also strategic planning, cultural adaptation, and a realistic assessment of AI's capabilities and limitations. There is also a need to address the disconnect between expectations set by AI's potential in the recent years of breakthroughs, set achievable targets, and track them to fruition. Organizations can set their own KPIs, and define what success means to them after moving to AIOps-led observability, and track key metrics to justify your means.
As we continue to explore and test AI's limits in IT, the key will be to leverage it for steady, meaningful improvements rather than chasing unattainable leaps. Strong leadership and a trained and motivated workforce that works with realistic expectations are key to moving AI-led observability to the next level.
Comments (0)