Taming alert chaos: How alarm overload leads to IT fatigue and how AIOps can fix
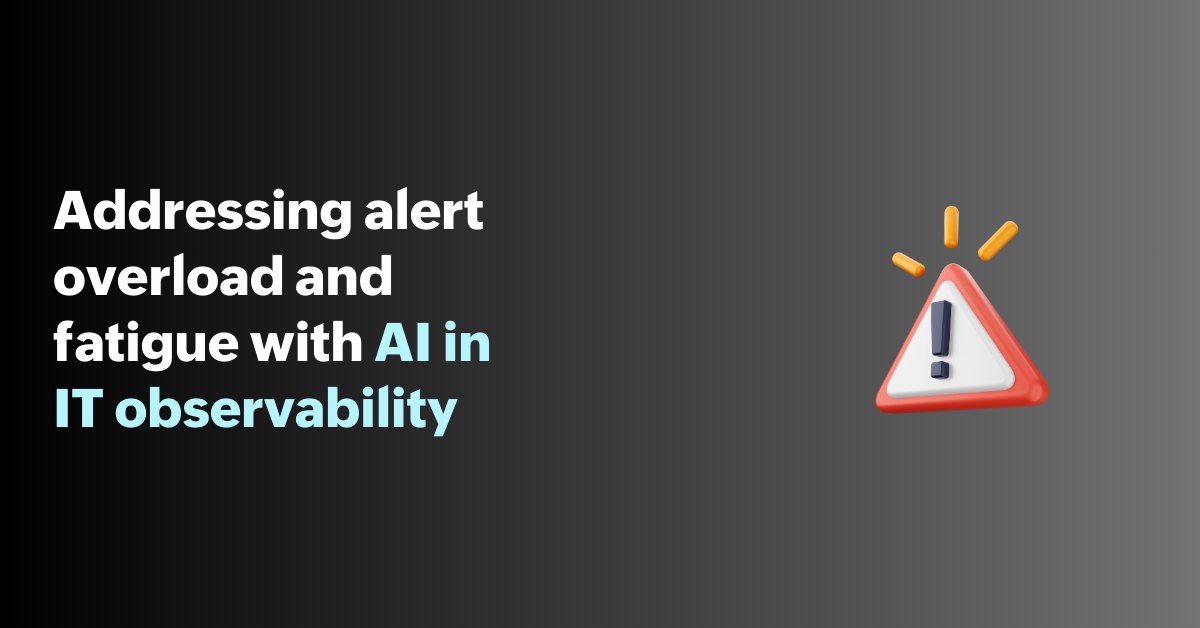
Data complexity increases every year. The three Vs of data—volume (the amount of data streaming in and out), velocity (the speed of generation, processing, and streaming), and variety (different forms ranging from structured databases and semi-structured XMLs to completely unstructured data as media files)—are also increasing in complexity.
Observability that makes sense of the data flow and function is becoming increasingly complex for DevOps and IT teams to deal with, and they cope with a bevy of monitoring tools to stay in the know. Often, alarms and alerts are set up with a maximalist mindset, where gross, fixed thresholds and values are entered in haste and are often uncorrected to match the changing times.
The result of unmanaged observability amid data chaos is a classic case of alarm overload or alert fatigue, an IT phenomenon that is not only a nuisance but a significant barrier to productivity and a threat to the team’s health and morale, ultimately impacting the system’s stability.
In this blog, let us see the anatomy of a typical case of an alert nightmare for a DevOps team and how IT observability powered by AIOps (the application of AI and ML in IT operations) brings in a revolutionary change in perspective for managing alerts. AIOps reduces noise, creating only the right alerts at the right time reliably in order for empowered IT teams to act with focus and conviction.
Imagine a normal day at the IT operations office. A critical production issue occurs. But your team members miss it because they have been swimming in hundreds of low-priority alerts. Worse, your on-call engineer calls it a day after the burnout of having been woken up multiple times for false positives over the last week. These are not hypothetical situations; they are daily realities for many IT teams, a consequence of unmanaged alerts.
The numbers are stark. A 2023 study of IT stakeholders by Enterprise Management Associates, an IT data management research firm, revealed that over 53% of all alerts are false alerts. This means wasted engineering hours chasing wild geese or mirages, resulting in less time available to tackle genuine incidents and thereby raking up operational costs, denting SLAs, and accelerating burnout.
The anatomy of an alerting nightmare
Let us assume an IT team handling a midsize application has set up a bunch of traditional-monitoring-tool-based alerts that are triggered based on static thresholds. This simplistic approach leads to several issues:
- False positives: When there is no double-checking to confirm an alert based on the current context and emerging trends, even transient issues like a temporary spike in CPU usage can end up triggering a large set of unnecessary alerts, leading to futile investigations.
- Contextless alerts: Data is meaningful only within a context. Without sufficient backing data that can be contextualized, it becomes hard for troubleshooters to ascertain the urgency or impact of alerts as they flow in. Without alert suppression, correlation, or grouping, useless alerts are sent in large numbers, clogging the view of the concerned personnel.
- Alert storms: When personnel are in an alert storm, their judgment often falters as a single issue can trigger multiple alerts across related services. This creates confusion and fatigue in the personnel, who often end up in a firefighting mode with no time to analyze patiently.
- A lack of automation: When alert rules are manually configured and not subject to rigorous, automatically correctable routines, they create alert storms every time there are unforeseen yet normal changes in the application’s behavior.
These problems compound, leading to a scenario where engineers are more focused on managing alerts than on innovating or maintaining system health.
The toll of IT fatigue
The consequences of alert chaos are profound and need to be understood well by all stakeholders to be systematically addressed and eliminated.
- Reduced productivity: Engineers spend large amounts of time sorting through alerts, diverting attention from strategic tasks.
- Burnout: The relentless alert barrage can lead to significant stress and fatigue, which reduces job satisfaction and could cause the attrition of talent.
- An increased mean time to resolution: Too many alerts slow down the process of identifying and addressing the actual problems, prolonging service disruptions.
- An erosion of trust: Over time, teams might start ignoring alerts, leading to them missing critical notifications.
What can AIOps do to transform alert management?
Here's where the application of AI and ML in IT operations helps redefine observability:
Enriched alerting
- Noise reduction: AI algorithms learn from historical data to filter out noise, dramatically reducing the volume of alerts. They distinguish between normal behavior fluctuations and actual anomalies, cutting down on false positives.
- Contextual enrichment: Alerts are enriched with contextual data like service dependencies, past incident correlations, and potential root causes, offering a clearer picture for quicker incident response.
- Predictive analytics: By analyzing trends, AI can predict issues before they manifest, allowing for preemptive action.
Streamlined incident response
- Automatic remediation: For known issues, AI can automatically execute predefined actions, such as service restarts, resource scaling via APIs, or rollbacks, minimizing human intervention.
- Incident orchestration: AI can automate the life cycle of incident management from ticket creation to remediation coordination, ensuring no step is missed.
Enhanced insights
- Anomaly detection: AI spots subtle deviations from normal operations that might be overlooked by traditional monitoring tools.
- Event correlation: AI connects events across sources to identify patterns and root causes, enabling faster anomaly detection.
- Root cause analysis: AI can sift through complex data relationships to find the source of issues, reducing the time spent on diagnostics.
The benefits of AI-driven observability
The advantages of integrating AI into IT observability are aplenty:
- A focus on what really matters: By intelligently filtering alerts, teams can focus on what truly matters for the company’s success.
- Faster incident response: Automation and intelligent workflows help teams address issues more swiftly.
- Increased productivity: With less time spent on alert management and more on deeper thinking and collaboration, teams can focus on innovation and strategy.
- Proactive IT operations: The predictive capabilities of AIOps help teams fix issues before they impact users, enhancing system reliability.
- Better customer experiences: Quicker resolutions and fewer disruptions lead to better service quality and customer satisfaction.
Empowering teams with ManageEngine Site24x7
At ManageEngine, we understand these challenges and have developed an AI-powered, full-stack IT observability platform to combat alert fatigue. Here’s how ManageEngine Site24x7 helps IT teams:
- Tackle alert fatigue: Our system uses AI to reduce false positives, providing a quieter, more focused alert environment. With as little as seven days of training data, Site24x7’s AI sets out to work and gets better as it learns your app’s patterns, becoming more accurate and remarkably dexterous in dealing with incidents to mark what matters and bring it to your attention.
- Boost productivity: AIOps can be used to automate routine tasks to help your team focus on what matters most, reducing stress and enhancing productivity.
- Empower personnel: With insights and automation now at their fingertips on centralized dashboards, your team members become more empowered to drive innovation and deliver exceptional service.
A few best practices on how to leverage AIOps in your observability strategy
- Start with clear objectives and KPIs. Know what to monitor, what to ignore, and what to automate.
- Invest time in training the AI system with historical data and train your team to handle data effectively.
- Implement gradually, starting with non-critical systems to avoid rude shocks or things spiraling out of control.
- Continuously refine and tune the IT stack based on feedback by listening to your team’s experience with AIOps in observability and its effectiveness.
- Maintain human oversight while trusting the AI's capabilities because the buck stops with your team. Therefore, have adequate checks and balances to audit your AIOps.
The journey from chaos to clarity with AIOps in IT observability
With the arrival of AIOps in IT observability, teams can focus better and rest assured with intelligent alerting, automation, and predictive analytics. With AIOps, organizations are able to not only recover from their current alert overloads and dark patterns in their systems but also build more resilient and efficient IT infrastructures. It's time to embrace AI-driven observability to ensure your team remains productive, stress-free, and empowered in the ever-changing IT landscape.
We invite DevOps and SRE teams to try ManageEngine Site24x7 to experience the power of AI on our IT observability platform that can help transform your IT operations, making them not only more efficient but also more rewarding for your engineers. Let us move from reacting to alerts to proactively managing your systems for a better tomorrow.
Comments (0)